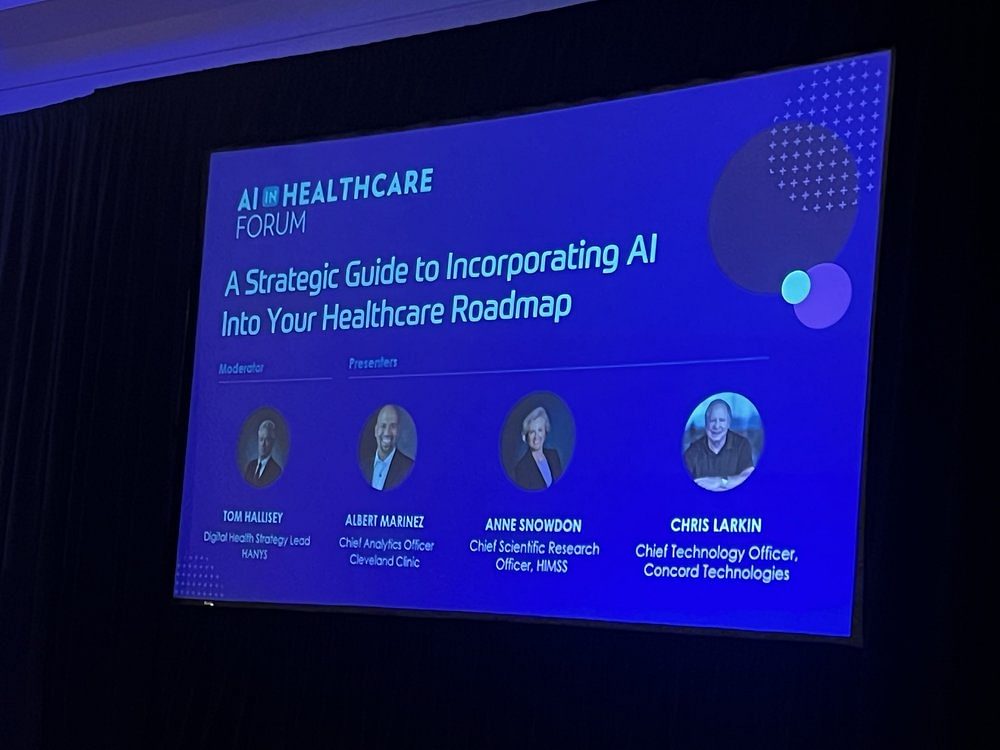
December was an action-packed month for the advancement of AI and ML in healthcare. From White House announcements to industry events and expert predictions, stakeholders from across our sector huddled to discuss AI’s promises, realities, and best practices. My main takeaway from December’s cacophony is that we’re all learning together and racing toward the same goal: responsible adoption of AI to improve health outcomes.
Across everything I heard, read, or attended, one healthcare stakeholder stands out as essential to the widespread adoption of AI in healthcare—our nation’s nursing professionals. Happy nurses lead to happy patients. And happy patients keep healthcare organizations (HCOs) in business.
Starting with this foundational truth, here are three best practices shared by speakers and attendees at the recent HIMSS AI in Healthcare Forum.
Meet with end users first. Anne Snowdon, CEO of SCAN Health and Chief Scientific Research Officer at HIMSS, reminded attendees that nurses can’t be given another task. Ask them to click one more box and they simply won’t do it. Systems must be designed to reduce nurses’ administrative burden and alert fatigue, not increase it.
Snowdon emphasized the need to check with end users before beginning any AI or ML initiative, including clinical and nonclinical teams. Think ahead to how an AI or ML solution will fit into the end-user workflow and accurately estimate what is needed for end-user training and change management to adopt the tool. Another speaker, Rachini Moosavi, Chief Analytics Officer, UNC Health, added that the hardest part of AI adoption will be integrating new systems into end-user workflows.
Sophia Henry, Clinical Consultant, Beckman Coulter Diagnostics and a 22-year nursing veteran, provided the following realities related to nurses and AI adoption:
-
Nurses need to focus on patients, not clicks.
-
Developers must first check with nurses to be sure new concepts will actually help them.
-
Nursing conversations must occur at the very beginning, not several weeks into an implementation.
-
Every detail of a nurse’s day-to-day workflow is important to understand.
-
Nursing knowledge can help IT companies build better products and systems.
And most important, Henry reiterated that AI systems aren’t designed to replace nurses. They are designed to support them.
Start with a business problem to solve, not the technology. Nearly every speaker during the two-day event stressed the importance of accurately defining a specific business problem before talking with vendors or evaluating technology solutions. Mid-revenue cycle, clinical documentation, prior authorization, and other operational processes were all identified as the best areas to begin the automation journey. For example, Atlantic Health System, Sanford Health, SSM Health, and Johns Hopkins all described success in automating clinician documentation and patient messages.
One speaker, Lars Maaloe, CTO, Corti, demonstrated how ambient clinical voice combines with AI to improve the clinician-patient dialogue in real time, relieve downstream administrative burdens, and build empathy through sentiment analysis.
Presenters suggested the following questions to determine your organization’s optimal starting point for AI or ML:
-
Is there a messy process that can be supported by AI or ML? And can we start with one aspect of that process?
-
How does the solution align with our organization’s strategic priorities?
-
What is the risk-value analysis?
-
How quickly can we demonstrate value and is it worth the risk?
-
Does the organization have clean, validated data to feed the AI solution from the start?
-
Do the end users see AI as a copilot or digital coworker to help them with their work?
-
Will the AI vendor be able to solve other problems in the future, not just the first use case?
-
Is the solution scalable?
Make sure your data is clean. Ann Cappellari, MD, CMIO, SSM Health, reminded attendees that “garbage in is still garbage out.” This legacy health IT system adage also applies to AI. Forrest Pascal, Principal, AI/ML Model Governance, Kaiser Permanente, reiterated this point by stating “Data may never be fully clean because humans are involved, but we can do our best to make sure the data is as unbiased as possible and we serve as good data stewards.”
Selection or construction of a large language model (LLM) is one of the first steps to consider. While LLMs will evolve over time, it is important to begin with a clean data set. Attendees were encouraged to seek out properly built and medically curated libraries to help ensure AI and ML outputs are valid and valuable to the end user. According to Severence MacLaughlin, CEO and Founder, DeLorean AI, there are variances in healthcare’s LLMs and outcomes shift as data changes. Organizations must avoid training LLMs with bad data.
Looking Ahead to AI in Healthcare
As Harvey Castro said during his opening keynote session, “There is too much on the line for us to get AI wrong in healthcare.” Our industry will learn over time just as our AI and ML solutions continually advance and improve.
As an example, healthcare organizations didn’t predict massive clinician burnout when HITECH was launched in 2009 and EHRs were universally implemented. Likewise, the long-term impacts of AI, ML, and other nascent technologies may not be realized for several years.
Harjinder Sandhu, CTO, Health and Life Sciences, Microsoft, concluded with an important reminder for all. “These systems are different from traditional health IT solutions. They need ongoing care, feeding, and training. We’re only one year into understanding how to use these systems in healthcare. The future holds much promise, and much responsibility.”
About the Author
Beth Friedman is a career healthcare professional and currently serves as Sr. Partner for FINN Partners. Based in Atlanta, Georgia, Beth is also co-host of FINN Voices podcast and a frequent author on health IT and digital health topics.